Admissibility of AI
The proliferation of artificial intelligence (AI) presents opportunities and challenges for litigation lawyers that extend into the courtroom. At its core, evidence law is framed by considerations of reliability.1 Because AI is a function of human selection of algorithms, the existence of deep fakes and bias and the viability of AI evidence are subject to challenge through expert testimony that addresses AI evidence reliability and authenticity, including bias, lack of testing for validity and reliability or consistency of output, function creep, and lack of explainability and transparency.2
Like many forms of electronically stored information (ESI), AI also is subject to potential alteration from human input, raising additional questions of reliability and admissibility that trial lawyers should not ignore.3 Moreover, admissibility decisions can be complex and especially difficult for judges, lawyers, and ultimately juries because there are no existing industry standards or specifications for expert opinions on algorithms and AI processes.4
The rules of evidence5 present multiple hurdles that must be cleared by the proponent of the evidence. Whenever ESI is offered as evidence, the following questions must be considered6:
Is the ESI relevant (does it have any tendency to make some fact that is of consequence to the litigation more or less probable than it otherwise would be)?
If relevant, is the ESI authentic (can the proponent show that the ESI is what it purports to be)?
If the ESI is offered for its substantive truth, is it hearsay and if so, is it covered by an applicable exception (Rules 803, 804, and 807)?
Is the form of the ESI that is being offered as evidence an original or duplicate under the original writing rule, or if not, is there secondary evidence to prove the content of the ESI?
Is the probative value of the ESI substantially outweighed by the danger of unfair prejudice or one of the other factors identified by Rule 403, such that it should be excluded despite its relevance?
There are some “big picture” evidentiary concepts to keep in mind when considering the admissibility of AI evidence. First, if a foundation cannot be established to show that the AI-powered technology produces accurate results, the evidence is unreliable and therefore has no relevance.7 By definition, unreliable evidence does not tend to prove or disprove facts that are of consequence to resolving a case or issue. However, determining the reliability of AI evidence depends on understanding how the applicable algorithm works.8 AI creators may have difficulty explaining how the algorithm was programmed or how it produces accurate results.9 Some of the main issues to anticipate in approaching admissibility questions concerning AI are summarized below.
Rule 104(a) and Conditional Admissibility
The relationship between Rule 104(a) and 104(b) can complicate the process by which ESI is admitted into evidence. Rule 104(a) states that “preliminary questions concerning the qualification of a person to be a witness, the existence of a privilege, or the admissibility of evidence shall be determined by the court.” Under Rule 104(b) the jury makes the factual findings determinative of admissibility. If the ruling on whether a document is an admission by a party opponent or a business record turns on contested facts, the admissibility of those facts would be determined by the judge under Rule 104(a). The admissibility of the document is for the jury to decide. Notably, proportionality is a mixed question of law and fact that often raises evidentiary issues regarding burden and benefit that are more suitable for the court to decide.10
Relevance
After conditional admissibility is sorted out, the starting place for any substantive evidentiary analysis is Rule 401, which defines evidence as relevant if it has “any tendency to make a fact more or less probable than it would be without the evidence” and “the fact is of consequence in determining the action.”11 Relevant evidence is inadmissible if its probative value is substantially outweighed by the danger of unfair prejudice, confusing the issues, misleading the fact finder, or wasting time or if the evidence is needlessly cumulative. In some situations, evidence regarding the use of AI may be prejudicial in the overall context of the case because it might mislead the jury on issues concerning the reliability and trustworthiness of such evidence. The question here is often weight, not admissibility.
Authentication
To authenticate AI technology, a proponent must show that the technology produces accurate, reliable results. The evidence can be established as authentic when it does what its proponents say it does. This means the following:
The accuracy of technical evidence has been verified by testing.
The methodology used to develop it has been published and is subject to review by others in the same field of science or technology.
The error rate associated with its use is not unacceptably high.
The standard testing methods and protocols have been followed.
The methodology used is generally accepted within the field of similar scientists or technologists.
Because the judge must act as the gatekeeper who determines whether the evidence can be considered by the jury, a party relying on AI evidence should provide sufficient evidence to authenticate the AI and prove its reliability.
Self-Authenticating AI
Rule 902(13) permits the self-authentication of certified records generated by an electronic system or process shown to produce an accurate result. Instead of calling one or more witnesses to establish the accuracy of the results of the AI technology, the party planning to introduce the AI evidence can prepare a certificate that meets the requirements of Rule 902(11). The certificate must be signed by witnesses with personal knowledge or technical expertise who would be called if the proponent of the AI evidence planned to authenticate it with witnesses.
Timothy D. Edwards, Wayne State 1989, is the owner of Edwards ESI LLC, Fitchburg, where he provides e-discovery consulting services and litigates construction, employment, and business disputes. As an adjunct lecturer at the University of Wisconsin Law School, he teaches electronic discovery, civil procedure, and pre-trial advocacy. He is a member of the State Bar of Wisconsin’s Intellectual Property & Technology Law Section and Labor & Employment Law Section. He is a Fellow of the Wisconsin Law Foundation.
Hayley Rich, U.W. 2024, is an attorney in the litigation practice at Godfrey & Kahn S.C., focusing primarily on complex civil litigation. While in law school, she was a clinical student with the Restorative Justice Project and represented the law school as a competitor at the 31st Willem C. Vis International Commercial Arbitration Moot in Vienna, Austria. She also competed in New York at the 31st Annual Duberstein Bankruptcy Moot Court Competition. She is a member of the State Bar of Wisconsin’s Young Lawyers Division.
In addition, Rule 901(b) provides 10 nonexclusive examples of how authentication of nontestimonial evidence can be accomplished. Rule 901(b)(1) permits the authentication of evidence through “[t]estimony that an item is what it is claimed to be.”
If this rule is used, then the witness must either meet the conditions of Rule 602 (requiring that witnesses have personal knowledge of the matters they testify about) or meet the qualification requirements of Rule 702 (that the witness has sufficient expertise to testify to a matter requiring scientific, technical, or specialized knowledge, experience, or training, in which case the witness may testify in the form of an opinion or otherwise).
AI and “Systems Integrity”
Rule 901(b)(9) is the second method of authentication for AI evidence. It permits authentication by producing evidence “describing a process or system and showing that it produces an accurate result.” In this regard, authenticating AI evidence using Rule 901(b)(9) will usually, if not always, be done the same way described above for Rule 901(b)(1) – by one or more witnesses with personal knowledge of the authenticating facts or one or more witnesses meeting the qualifications of Rule 702.
Authentication Through Expert Testimony
Expert testimony can be used to authenticate AI. Under Rule 901(b)(1) or 901(b)(9),
an expert on authentication must either 1) have personal knowledge of the authenticating facts, or 2) qualify as an expert who is permitted to incorporate into their testimony information from sufficiently reliable sources beyond their own personal knowledge.12
AI technology is a technical scientific issue, so expert testimony in support of specific AI admissibility can be challenged subject to the Daubert standards. In the seminal article Artificial Intelligence as Evidence, Judge Paul Grimm offered the following potential inquiry to test the reliability and validity of AI evidence under Daubert and to meet the authentication requirements:
What problem was the AI created to solve?
How was the AI developed, and by whom? Who wrote the code?
Was the validity and reliability of the AI sufficiently tested?
Is how the AI operates “explainable” so that it can be understood by counsel, the court, and the jury?
What is the risk of harm if AI evidence of uncertain trustworthiness is admitted?13
One of the more formidable issues with AI is the cost of presenting or challenging the evidence because of the requirement of expert testimony to authenticate the evidence. There is a danger that the introduction of and opposition to AI evidence will devolve into an expensive diversion with dueling experts.14
Hearsay
The first question in analyzing hearsay in the AI context is whether an AI entity can be considered a declarant. Recent legal, academic, and scientific scholarly works have suggested that AI technology will continue to develop and eventually result in person-like AI entities.15 But because the hearsay rule and its exceptions were designed with human declarants in mind, one can imagine that an AI entity’s statement, even if made by an AI entity that is fully autonomous and essentially indistinguishable from a human, might not neatly fit under any hearsay exceptions.16
For instance, can an AI entity have a state of mind or a present-sense impression?17 Will an AI entity understand the potential consequences when it makes a statement against its own interest?18 Perhaps the most advanced sentient versions of future AI entities, if they ever exist, will possess these qualities, but it is far less clear that the more probable future AI entities will.19 Though many of the hearsay exceptions seem to presuppose the existence of certain qualities such as a state of mind or a self-interest, the rules do not explicitly impose such a requirement or explicitly state that only a human is capable of having those qualities.
Expert Testimony to Support or Dispute Admissibility
A party can authenticate, establish relevance, and enhance the reliability of AI by demonstrating the accuracy of its results through the proper use of expert testimony. As a starting point, Rule 702 requires that expert testimony be based on sufficient facts and reliable methodology and reliably applied to the facts of the case. Under Rule 702, the trial court serves as a “gatekeeper” to prevent unreliable and irrelevant scientific testimony from entering the courtroom.
Trial courts must use four nonexclusive factors to determine the reliability of expert testimony: 1) whether the “scientific knowledge ... can be (and has been) tested”; 2) whether “the theory or technique has been subjected to peer review and publication”; 3) “the known or potential rate of error”; and 4) “general acceptance.”20 These guidelines help the trial court ensure that scientific testimony or evidence, including AI, is relevant and reliable.
In his article, Frank Griffin noted how the Daubert factors entwine with AI evidence. He stated, “Importantly, AI manufacturers will have decisive advantages in all four of [the Daubert factors]. First, the AI companies will likely be the ones doing the scientific testing, which may bias research outcomes. Second, peer review and publication will likely be performed by AI scientists working for companies, again introducing bias. Third, any known or potential error rate will likely be discovered by AI companies, which may limit disclosure. Fourth, general acceptance will be up to AI scientists working for AI companies, which may limit the field of witnesses available to testify in support or objection to its admissibility.”21
Conclusion
AI is dynamic technology that raises unique questions regarding the preservation, production, and admissibility of ESI. Discovery issues involving AI typically center around proportionality, which requires the trial court to balance the potential relevance of the information against several other factors, including cost. The biggest challenges in admitting AI, like other ESI, are authentication and, in some cases, reliability – issues that often are addressed by experts.
Lawyers who understand AI and target specific data for appropriate reasons will be in the best position to discover important information by using specific discovery requests, bound by subject matter and temporal scope, that seek material information that is not prejudicial, cumulative, or confusing. Lawyers who navigate through discovery with a view toward admissibility will be in the best position to authenticate and establish the reliability and relevance of proffered evidence by laying the appropriate foundation. Parties opposing the discovery and admission of AI data should be aware of the inherent bias and related pressure points that call its reliability into question.
The possibilities are enticing for creative litigation attorneys who seek to harness the power of this dynamic, developing technology and to contribute to new developments in this emerging area of the law.
Also of Interest
The Source for Wisconsin Discovery Law
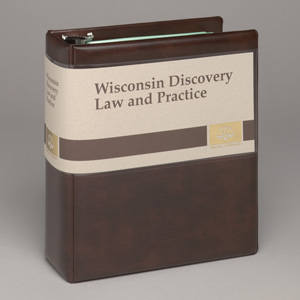
With Wisconsin Discovery Law and Practice, attorneys can tap into the knowledge and experience of some of Wisconsin’s most successful litigators and become exceptional in the realm of discovery. Learn more about the scope of discovery; the functions, advantages, and disadvantages of formal and informal discovery; how to modify discovery procedures; the effects of the general requirement of relevance on actions and cases; the role of privileges and immunities in limiting the nature and extent of discovery; and the application of the doctrine of waiver and forfeiture in a discovery setting.
Discovery Law and Practice is packed with hundreds of useful examples, practice tips, instructions, and sample questions addressing the key facets of discovery, beginning with initial discovery and including interrogatories; inspection of documents, places, and things; and judicial supervision and enforcement. The book’s 10 chapters, sample forms, and thorough index will help you clarify discovery law in Wisconsin.
Wisconsin Discovery Law and Practice is an efficient guide to the discovery process in Wisconsin. For those who spend only part of their time in litigation, the book will become a valuable reference as you work your way through your case. For those whose entire practice deals with litigation, the book will become your guiding authority for discovery in Wisconsin.
https://marketplace.wisbar.org/products?pid=AK0042
Endnotes
1 Daniel D. Blinka, Ethics, Evidence, and the Modern Adversary Trial, 19 Geo. J. Legal Ethics 1, 7 (2006), https://scholarship.law.marquette.edu/facpub/290/.
2 Paul W. Grimm et al., Artificial Intelligence as Evidence, 19 Nw. J. Tech. & Intell. Prop. 9, 97 (2021), https://scholarlycommons.law.northwestern.edu/cgi/viewcontent.cgi?article=1349&context=njtip.
3 Id. at 45.
4 1 Florida Civil Trial Practice § 13.3 (2024).
5 In this article, the “rules of evidence” means the Federal Rules of Evidence and the Wisconsin Rules of Evidence, which are similar in many respects. SeeRacine Educ. Ass’n v. Board of Educ. for Racine Sch. Dist., 129 Wis. 2d 319, 326, 385 N.W.2d 510 (Ct. App. 1986) (“Whenever we are construing a state statute patterned after a federal rule, federal case law is persuasive authority.”). References in this article to “Rule XXX” are to a rule in the Federal Rules of Evidence. The Wisconsin Rules of Evidence are in Wis. Stat. chapters 901-911.
6 Loraine v. Markel Am. Ins. Co., 241 F.R.D. 534, 538 (D. Maryland 2007).
7 The Sedona Conference et al., The Sedona Conference Commentary on ESI Evidence & Admissibility, Second Edition – A Project of The Sedona Conference Working Group on Electronic Document Retention and Production, 22 Sedona Conf. J. 83, 186 (2021).
8 Id.
9 Id.
10 Ralph C. Losey, Predictive Coding and the Proportionality Doctrine: A Marriage Made in Big Data, 26 Regent U.L. Rev. 7, 44 (2014), https://www.regent.edu/acad/schlaw/student_life/studentorgs/lawreview/docs/issues/v26n1/8_Losey_vol_26_1.pdf.
11 Fed. R. Evid. 401.
12 See Fed. R. Evid. 602, 702, 703; Wis. Stat. § 907.02(1).
13 1 LN Practice Guide: Florida E-Discovery & Evidence 15.16 (2024); see Grimm, supra note 2, at 97-105.
14 1 LN Practice Guide, supra note 13, at 15.16.
15 Jess Hutto-Schultz, Dicitur Ex Machina: Artificial Intelligence and the Hearsay Rule, 27 Geo. Mason L. Rev. 683, 686 (2020).
16 Id. at 715.
17 Id.
18 Id.
19 Id.
20 See Daubert v. Merrell Dow Pharm. Inc., 509 U.S. 579, 593-94 (1993).
21 Frank Griffin, Artificial Intelligence and Liability in Health Care, 31 Health Matrix 65, 91 (2021).
» Cite this article: 97 Wis. Law. 8-12 (December 2024).